Data-driven decision making is a critical aspect of modern business strategy, enabling organizations to harness the power of data to make informed and strategic choices. By leveraging data analytics and insights, businesses can gain a competitive edge, drive innovation, and enhance customer experiences. This article explores the key components of data-driven decision making, its benefits, challenges, strategies for implementation, case studies, and the future of this evolving practice.
Key Takeaways
- Data-driven decision making empowers organizations to make informed and strategic choices based on data analytics and insights.
- The benefits of data-driven decisions include improved accuracy and efficiency, enhanced customer experiences, and driving innovation and competitive advantage.
- Challenges in data-driven decision making include overcoming data quality issues, navigating privacy and ethical concerns, and building the right team for data analytics.
- Strategies for implementing data-driven practices involve establishing clear goals and objectives, investing in the right tools and technologies, and fostering collaboration across departments.
- The future of data-driven decision making lies in predictive analytics, machine learning, artificial intelligence, and the continuous pursuit of learning and adaptation.
Understanding Data-Driven Decision Making
Defining Data-Driven Decision Making
At its core, data-driven decision making (DDDM) is a process that involves collecting data, extracting patterns and insights, and using these findings to guide strategic business decisions. Unlike intuition-based decisions, DDDM relies on data analysis to predict outcomes, optimize operations, and mitigate risks.
Data-driven organizations often follow a structured approach to decision making. This includes identifying key performance indicators (KPIs), gathering relevant data, and applying analytical models to interpret the results. The insights gained from this process inform actions that are aligned with the company's strategic goals.
- Identify KPIs
- Gather data
- Analyze and interpret
- Inform strategic actions
Embracing a data-driven approach can significantly enhance decision-making quality, but it requires a commitment to data accuracy and the development of robust analytics capabilities.
The Evolution of Data in Business Strategy
The evolution of data in business strategy has transformed the way organizations operate. Data-driven decision making has become a cornerstone of success, enabling companies to leverage insights for strategic planning, resource allocation, and performance evaluation. This shift has led to a more agile and adaptive approach to business, where decisions are informed by real-time data and predictive analytics.
Implementing a table for presenting structured, quantitative data can provide a clear visualization of key metrics, trends, and performance indicators. This allows stakeholders to quickly grasp complex data and make informed decisions based on the presented information.
Tip: When using a table, ensure that the data is relevant, concise, and easily understandable for all stakeholders.
Key Components of a Data-Driven Culture
Cultivating a data-driven culture within an organization goes beyond merely collecting and analyzing data. It requires a foundational shift in how decisions are made and how success is measured. At the heart of this culture are several key components:
- Leadership commitment to data-driven principles is essential. Leaders must champion the use of data and analytics in decision-making processes.
- A strategic alignment of data initiatives with business goals ensures that efforts are not in silos but contribute to the overall objectives of the company.
- Robust data infrastructure and tools provide the necessary backbone for storing, processing, and analyzing large volumes of data.
- Data literacy among employees empowers them to understand and utilize data effectively in their roles.
- A strong governance framework ensures data quality and integrity, which are critical for accurate analysis.
Tip: Encourage a culture of experimentation and learning. Data-driven decision making often involves testing hypotheses and learning from the outcomes, whether they confirm or challenge initial assumptions.
These components are interdependent, and their collective strength determines the robustness of a data-driven culture. Without a commitment from leadership or a lack of data literacy, the potential of data to transform business operations and strategy can be severely limited.
The Benefits of Data-Driven Decisions
Improving Accuracy and Efficiency
In the realm of data-driven decision making, accuracy and efficiency are paramount. By leveraging high-quality data, organizations can significantly reduce the margin of error in their decision-making processes. This precision, in turn, leads to more efficient operations and resource allocation. For example, predictive analytics can forecast demand, optimizing inventory management and reducing waste.
Automation plays a critical role in enhancing efficiency. It eliminates manual tasks, speeds up data processing, and ensures consistent application of business rules. Here's how automation impacts various business functions:
- Marketing: Tailoring campaigns to customer behavior
- Sales: Streamlining lead qualification and follow-up
- Operations: Improving supply chain responsiveness
- HR: Simplifying recruitment and onboarding processes
Tip: Always validate the data sources and algorithms used in automation to maintain the integrity of decision-making.
The integration of machine learning and other advanced technologies further refines the accuracy of predictive models, enabling businesses to anticipate market trends and customer needs with remarkable precision. As a result, companies not only save time and money but also gain a competitive edge by responding proactively to emerging opportunities.
Enhancing Customer Experiences
In the age of digital commerce, customer experience has become a pivotal aspect of business success. Companies that leverage data to understand and enhance customer interactions often see a significant improvement in customer satisfaction and loyalty. Personalization, for instance, is a powerful tool that drives not only revenue but also customer loyalty and competitive advantage. By analyzing customer data, businesses can tailor their communication, offers, and services to meet the unique needs of each individual, fostering a sense of customer intimacy that is crucial for long-term success.
Automated CRM touchpoints and AI-enhanced A/B testing are examples of how data can be utilized to refine the customer journey. Implementing a referral program can turn satisfied customers into brand ambassadors, while direct-to-consumer (DTC) advertising can be optimized to increase customer lifetime value. These strategies, when informed by robust data analysis, can transform the customer experience from satisfactory to exceptional.
Tip: Always consider the customer's perspective when analyzing data. Insights that improve convenience, speed, and relevance are often the most impactful in enhancing the customer experience.
Driving Innovation and Competitive Advantage
In the realm of business, innovation and competitive advantage are often the byproducts of a robust data-driven approach. Companies that leverage data effectively can identify new market opportunities, optimize their products and services, and outpace competitors. By analyzing trends and patterns, businesses can predict customer needs and craft innovative solutions that resonate with their target audience.
Data-driven insights also enable organizations to refine their strategies in real-time, ensuring that they remain agile in a rapidly changing market. This agility is crucial for sustaining long-term growth and maintaining a competitive edge. For instance, by harnessing the power of data analytics, a company can streamline its operations, reduce waste, and increase productivity, all of which contribute to a stronger market position.
To maintain a lead in innovation, it is essential to foster a culture that values data literacy and encourages the exploration of data for new ideas.
While the pursuit of innovation through data is compelling, it is not without its challenges. Companies must navigate the complexities of data management and analysis, ensuring that they have the right tools and expertise to extract meaningful insights. The table below illustrates some key factors that influence the success of data-driven innovation:
Factor
Description
Data Quality
High-quality data is the foundation of accurate insights.
Analytical Tools
Advanced tools are necessary for sophisticated data analysis.
Expertise
Skilled analysts are crucial for interpreting data correctly.
Culture
An organizational culture that embraces data is vital for ongoing innovation.
Challenges and Considerations
Overcoming Data Quality Issues
High-quality data is the foundation of any data-driven decision-making process. However, organizations often face challenges in ensuring the accuracy, completeness, and reliability of their data. To overcome these issues, it's crucial to establish rigorous data governance practices. This includes the implementation of standards, policies, and procedures that govern the collection, storage, and use of data.
Data cleansing is another vital step in enhancing data quality. It involves identifying and correcting errors, inconsistencies, and duplications in datasets. Regular audits and validation checks can help maintain the integrity of data over time. Additionally, investing in robust data integration tools can streamline the consolidation of data from various sources, ensuring a unified and accurate view.
Tip: Always validate new data sources before integrating them into your decision-making framework to prevent the propagation of errors.
Here are some key actions to consider:
- Establishing a cross-functional data governance team
- Defining clear data quality metrics
- Implementing automated data cleansing solutions
- Conducting regular data quality assessments
- Encouraging a culture of data quality awareness among employees
Navigating Privacy and Ethical Concerns
In the realm of data-driven decision making, privacy and ethical concerns stand as significant challenges that organizations must navigate with care. The use of personal data, especially in sensitive sectors like healthcare or finance, requires a delicate balance between leveraging data for business insights and respecting individual privacy rights.
Transparency in data collection and use is critical. Companies should clearly communicate to their customers what data is being collected, how it is being used, and who has access to it. This not only builds trust but also aligns with regulatory requirements such as GDPR and CCPA.
Here are some key principles to consider:
- Ensuring compliance with data protection laws
- Establishing clear data governance policies
- Regularly auditing data usage and security measures
- Providing customers with control over their data
Tip: Always prioritize user consent and data minimization to maintain ethical standards and avoid potential legal pitfalls.
As organizations strive to harness the power of data, they must also invest in the right tools and training to safeguard against breaches and misuse. The goal is to create a data ecosystem that is both powerful and responsible, ensuring that data-driven growth does not come at the cost of consumer trust or ethical integrity.
Building the Right Team for Data Analytics
Building the right team for data analytics is crucial for the success of data-driven decision making. It requires a combination of technical expertise, domain knowledge, and strong communication skills. Collaboration across departments is essential to ensure that data insights are effectively utilized throughout the organization.
When assembling a data analytics team, it's important to consider the diverse skill sets required. This may include data scientists, data engineers, business analysts, and domain experts. Diversity in skill sets and perspectives can lead to more comprehensive and innovative solutions.
Tips for Building the Right Data Analytics Team:
- Clearly define roles and responsibilities within the team.
- Foster a culture of continuous learning and knowledge sharing.
- Encourage cross-functional collaboration to leverage diverse expertise.
Building a cohesive and well-rounded data analytics team is a strategic investment that can drive the success of data-driven decision making across the organization.
Strategies for Implementing Data-Driven Practices
Establishing Clear Goals and Objectives
In the journey towards data-driven excellence, the first and perhaps most critical step is establishing clear goals and objectives. Without a well-defined destination, the path can quickly become muddled, leading to wasted resources and lost opportunities. Goals should be specific, measurable, achievable, relevant, and time-bound (SMART), providing a framework for what success looks like.
Setting objectives is not just about the end result; it's about creating a roadmap for the journey. This involves identifying key performance indicators (KPIs) that will serve as milestones along the way. A common pitfall is setting too many goals, which can dilute focus and resources. Prioritization is essential to ensure that the most impactful areas are addressed first.
Tip: Always align data-driven goals with the overall business strategy to ensure that every effort contributes to the broader company vision.
A structured approach to goal-setting can be facilitated by a simple table:
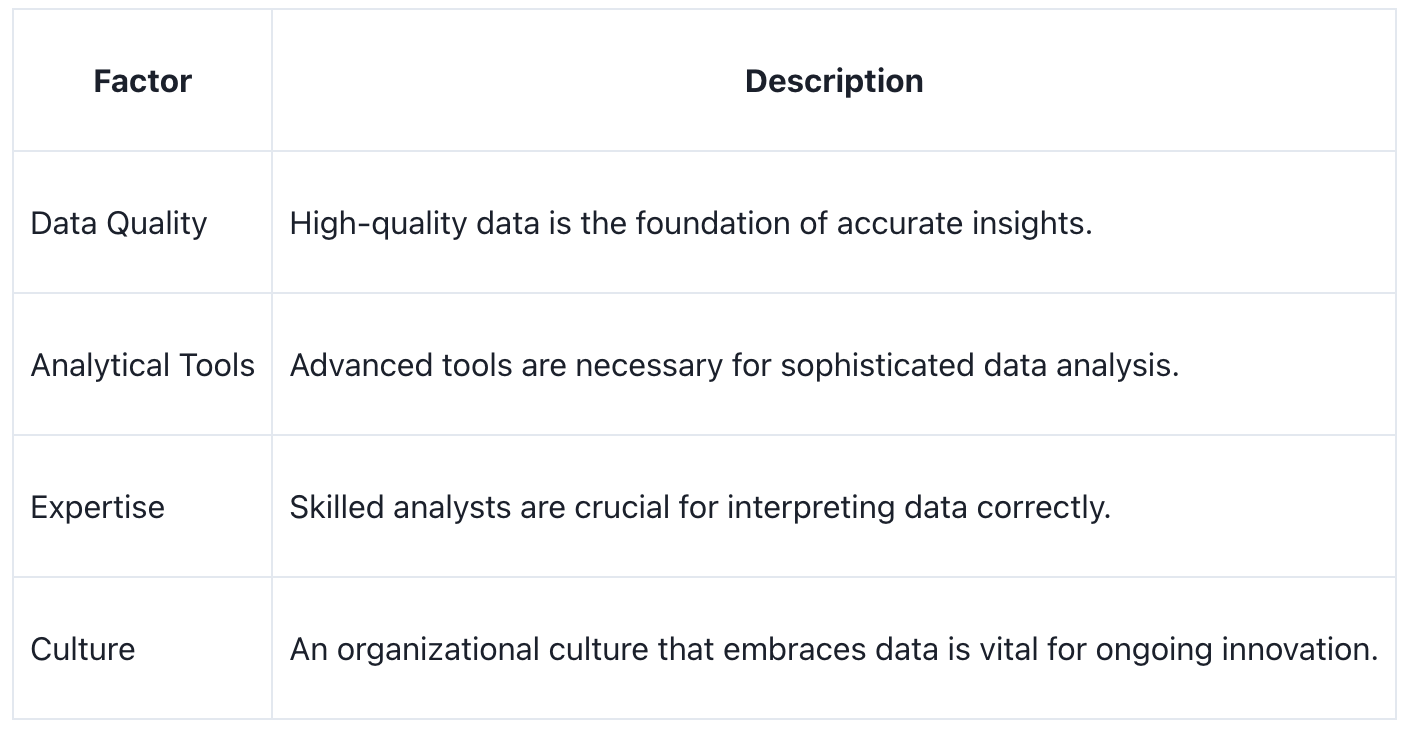
By clearly defining what you want to achieve, you lay the groundwork for a data-driven culture that is focused, strategic, and primed for success.
Investing in the Right Tools and Technologies
When investing in the right tools and technologies for data-driven practices, it's crucial to prioritize integration. Seamless integration across platforms and systems ensures efficient data flow and accessibility. Additionally, consider the following key factors:
- Compatibility with existing infrastructure
- Scalability and flexibility
- Security and compliance
For structured, quantitative data, implementing a Markdown table can effectively present comparisons, metrics, or specifications. This allows for clear visualization and easy reference. When dealing with less structured content, such as steps or related items, a bulleted or numbered list can provide a concise and organized format for conveying information.
Tip: Prioritize tools and technologies that offer robust support for data governance and data security, as these are critical for maintaining the integrity and confidentiality of data.
Fostering Collaboration Across Departments
Fostering collaboration across departments is crucial for the success of data-driven practices. It enables the sharing of insights, best practices, and resources, leading to a more cohesive and informed decision-making process. By breaking down silos and encouraging open communication, organizations can leverage the collective expertise of different teams to drive innovation and problem-solving. This collaborative approach also fosters a culture of transparency and accountability, aligning everyone with the common goal of leveraging data for strategic decision making.
Case Studies: Success Stories and Lessons Learned
Industry Leaders Who Excel at Data-Driven Decisions
When it comes to data-driven decision making, industry leaders set the standard for leveraging data to drive business success. These companies prioritize data collection, analysis, and interpretation to inform strategic initiatives and operational improvements. The commitment to data-driven practices is evident in their consistent track record of informed decision making and sustainable growth. This dedication to data-driven decision making has positioned these companies as trailblazers in their respective industries, setting a benchmark for others to follow.
Analyzing Failures: What Can Go Wrong?
When venturing into the realm of data-driven decision making, companies may encounter a series of pitfalls that can lead to significant setbacks. One common issue is the misinterpretation of data, which can result in misguided strategies and wasted resources. It's crucial to have a team that not only understands how to analyze data but also has the expertise to draw the correct conclusions.
Another critical aspect is ensuring data integrity. Inaccurate or incomplete data can skew results and lead to poor decision making. Companies must establish rigorous data governance practices to maintain the quality and reliability of their data.
Here are some key factors that contribute to failures in data-driven decision making:
- Lack of clear objectives
- Inadequate data infrastructure
- Insufficient data literacy among staff
- Failure to integrate data insights into the decision-making process
Tip: Always validate your data sources and double-check your analytics for consistency before making major decisions.
Adapting to Change: How Companies Pivot with Data
In an ever-evolving marketplace, the ability to adapt quickly to change is a hallmark of successful companies. Data plays a pivotal role in this agility, providing the insights necessary to make informed decisions swiftly. Companies that excel in using data to pivot can identify trends, anticipate market shifts, and respond to customer needs with precision.
To pivot effectively, businesses often follow a series of steps:
- Monitor real-time data to detect early signs of change.
- Analyze the impact of potential shifts on different segments of the business.
- Develop scenarios and model outcomes based on various data-driven strategies.
- Implement changes swiftly, while continuing to measure and adjust strategies based on new data.
Tip: Always keep your data infrastructure flexible to accommodate rapid changes in strategy.
By embracing a culture that values data and its insights, organizations can turn potential disruptions into opportunities for growth. It's not just about having data, but about having the right systems and processes in place to make that data actionable.
The Future of Data-Driven Decision Making
Predictive Analytics and Machine Learning
The advent of predictive analytics and machine learning has revolutionized the way businesses forecast trends and behaviors. By analyzing historical data, these technologies can identify patterns and predict future outcomes with a high degree of accuracy. This foresight enables companies to make proactive decisions, rather than reactive ones, giving them a significant edge in the marketplace.
Predictive analytics is not just about forecasting; it's also about understanding the probability of various scenarios. This allows businesses to prepare for multiple potential futures, ensuring resilience and adaptability. Machine learning algorithms continually refine their predictions, learning from new data to improve over time.
Tip: Start small with predictive analytics by focusing on a single business question that can be answered with data. This approach helps in gaining quick wins and building organizational confidence in data-driven methods.
The implementation of these technologies requires careful consideration of the data's quality and the potential biases inherent in the algorithms. It's essential to have a robust framework for data governance and ethics to ensure that predictions are fair and equitable.
The Role of Artificial Intelligence
Artificial intelligence (AI) plays a pivotal role in data-driven decision making, offering advanced capabilities for processing and analyzing large datasets. It enables organizations to extract valuable insights from complex data, driving informed decision making and strategic planning. Implementing AI-powered solutions requires a combination of technical expertise and domain knowledge, ensuring that the algorithms are trained on relevant data to produce accurate and actionable results. Leveraging AI in data-driven practices can lead to significant improvements in operational efficiency, predictive modeling, and strategic decision making. Organizations that embrace AI are better positioned to adapt to dynamic market conditions and gain a competitive edge through data-driven innovation and agility.
Staying Ahead: Continuous Learning and Adaptation
In the realm of data-driven decision making, the ability to stay ahead hinges on a commitment to continuous learning and adaptation. As the landscape of data and analytics evolves, organizations must remain agile, constantly updating their knowledge and competencies to leverage new insights and technologies.
To maintain a competitive edge, companies should foster a culture of curiosity and lifelong learning among their employees. This can be achieved through regular training sessions, workshops, and the encouragement of cross-departmental knowledge sharing. Additionally, staying informed about industry trends and emerging tools can help businesses anticipate changes and adapt swiftly.
Tip: Always be prepared to question existing assumptions and embrace new methodologies. The willingness to experiment and learn from both successes and failures is crucial for long-term growth.
Investing in advanced analytics and machine learning platforms can also play a significant role in staying ahead. These technologies not only provide deeper insights but also enable predictive capabilities that can inform future strategies. It's not just about having the data but being able to act on it effectively and efficiently.
Conclusion
In conclusion, the power of data-driven decision making cannot be overstated. By leveraging data, organizations can gain valuable insights and make informed decisions that drive success. Embracing a data-driven approach is essential in today's rapidly evolving business landscape, and it empowers businesses to adapt, innovate, and thrive.
Frequently Asked Questions
What is data-driven decision making?
Data-driven decision making is the process of making informed business decisions based on data analysis and insights rather than intuition or gut feeling.
Why is data-driven decision making important?
Data-driven decision making is important because it leads to more accurate and efficient decision making, enhances customer experiences, and drives innovation and competitive advantage.
What are the challenges of data-driven decision making?
Challenges of data-driven decision making include overcoming data quality issues, navigating privacy and ethical concerns, and building the right team for data analytics.
How can organizations implement data-driven practices?
Organizations can implement data-driven practices by establishing clear goals and objectives, investing in the right tools and technologies, and fostering collaboration across departments.
What are some success stories of data-driven decision making?
Industry leaders who excel at data-driven decisions serve as success stories, while analyzing failures and adapting to change also provide valuable lessons.
What does the future hold for data-driven decision making?
The future of data-driven decision making includes predictive analytics and machine learning, the role of artificial intelligence, and the importance of continuous learning and adaptation to stay ahead.